The surge in retail lending, including personal loans, consumer durable loans and vehicle finance, has emphasized the importance of robust monitoring mechanisms to mitigate risks and ensure asset quality. A well-designed Early Warning System (EWS) is crucial to proactively identify potential defaults, offering timely interventions to mitigate risks. This article outlines a structured approach to delivering an EWS for retail assets, focusing on constructing a scorecard and integrating essential data sources (LOS, LMS, Collection etc.).
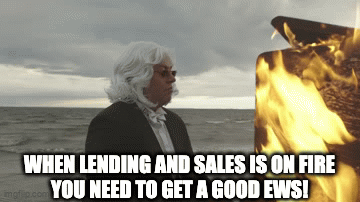
1. Constructing a Scorecard: The Foundation of EWS
A scorecard lies at the heart of an EWS, translating complex data into actionable risk scores. Here's how to build it:
a. Identify Key Risk Parameters
For retail assets, parameters should reflect the borrower's financial behavior, macroeconomic indicators, and portfolio health. Common factors include:
Payment Behavior: Missed or delayed payments, changes in repayment amounts.
Borrower Profile Changes: Variations in employment, income stability, or credit utilization.
Loan Attributes: Loan-to-value ratio, tenure, interest rate trends.
b. Assign Weights to Parameters
Not all parameters contribute equally to risk. A scoring model assigns weights based on historical data and predictive analytics. For instance:
Payment Behavior: 40%
Credit Score Trends: 30%
Macroeconomic Indicators: 20%
External Factors (e.g., News, City, Sector Updates): 10%
c. Apply Dynamic Weighting
Dynamic weightings allow the model to adapt to external shocks (e.g., economic slowdowns or sectoral crises). For example, during an industry downturn, sectoral performance may weigh more heavily than payment behavior. Drona also offers the ability to leverage ML models to compute the weights dynamically.
d. Calibrate and Validate
Test the scorecard against historical defaults to ensure predictive accuracy. Periodic recalibration ensures it reflects current market conditions.
2. Parameters
Retail asset monitoring requires a mix of borrower-level and macroeconomic parameters.
a. Borrower-Level Parameters
Credit Score Dynamics: Trends in creditworthiness over time.
Debt-to-Income Ratio: Increased ratios can indicate financial strain.
Employment and Income Trends: Especially critical for personal loans and vehicle finance.
b. Loan-Specific Parameters
Loan-to-Value (LTV) Ratios: Key for housing loans and vehicle finance.
Overdue Days: Early signs of potential delinquency.
Repayment History: Patterns in repayment consistency or amounts.
c. External Parameters
Macroeconomic Trends: Unemployment rates, inflation, interest rate hikes.
Sectoral and Regional Performance: Housing price indices for housing loans, auto industry reports for vehicle finance.
News and Events: Labor unrest, regulatory changes, or disasters affecting specific geographies or industries.
3. Integrating External Data Sources
The value of an EWS is amplified by integrating diverse data sources beyond internal banking systems.
a. Real-Time News Feeds
Subscribe to news APIs or data aggregators to flag developments like layoffs, regulatory actions, or sector-specific downturns.
Example: News of a major employer's closure in a region can indicate risks for personal and housing loan borrowers in that area.
b. Housing and Auto Platforms
For housing loans, monitor price trends from real estate platforms and sector reports on construction activity or demand-supply mismatches.
For vehicle finance, track vehicle sales trends, resale values, and fuel price movements.
c. Regional and Sectoral Reports
Integrate reports on GDP growth, urbanization rates, or industry performance for localized insights.
Example: A report showing stagnation in rural demand could flag risks in personal loans for rural borrowers.
4. Real-Time Alerts and Monitoring
A robust EWS should provide real-time alerts to identify potential red flags. Use a combination of:
Threshold Triggers: Automatic alerts when a borrower’s risk score exceeds a set limit.
Periodic Reviews: Quarterly reviews of sectoral and geographic data to recalibrate thresholds.
Case Management: A module to investigate flagged accounts, track resolution actions, and document findings.
5. The Role of Technology in EWS Implementation
a. Machine Learning Models
ML models can refine scorecards by detecting non-linear relationships and adjusting for unseen patterns.
Use supervised learning for historical data analysis.
Incorporate unsupervised learning to flag anomalies.
b. Cloud-Based Platforms
A centralized cloud platform facilitates seamless integration of internal and external data, enabling real-time updates and analytics.
c. Visualization ToolsDashboards with interactive visualizations enable stakeholders to identify trends and anomalies quickly.
Conclusion
Developing an Early Warning System for retail assets requires a careful blend of internal insights, external data, and technology. A well-calibrated scorecard, combined with diverse data inputs and real-time monitoring, empowers lenders to mitigate risks proactively. As the retail lending landscape evolves, adopting such systems will be instrumental in safeguarding portfolios and ensuring sustainable growth.
Drona offers a leading Analytics and Decisioning platform to construct scorecards based on an array of data sources (Internal and External) as well as ability to leverage ML models like Probability of Default (PD), Loss Given Default (LGD) etc. Build a comprehensive Portfolio Analytics Platform starting with Early Warning Systems from Drona.
Comments